The goal of this project is to experimentally quantify the tearing stability of tokamak equilibria using physics-informed machine learning (ML), for the purpose of finding potential tearing-free pilot-plant regimes.
Tearing modes are instances of magnetic reconnection that grow into magnetic islands. They have resonant modal structure, and are driven by free energy in the current profile, pressure and temperature gradients. It is unlikely that a tokamak pilot-plant can operate with them present, as tearing modes degrade confinement, and lead to locked modes and disruptions.
While the high temperatures and densities required for net fusion energy gain generally lead pilot-plant equilibria towards tearing instability, there may be regions of the design-space where we can achieve suitably high energy gain without seeding tearing modes. This project is about finding these regions and quantifying their reliability through data-base analysis.
The chosen approach is to proceed from linear tearing analysis in cylindrical geometry, to full toroidal treatment with poloidal mode coupling present. The pilot-plant design-space is being explored using Monte-Carlo sampling of profiles and shaping parameters, to predict new regions of increased tearing-stability. These stability predictions are being verified via resistive MHD simulations in M3D-C1. Nonlinear tearing analysis will be included in the form of the modified Rutherford equation (MRE), with interest in extending the nonlinear treatment to include poloidal mode coupling.
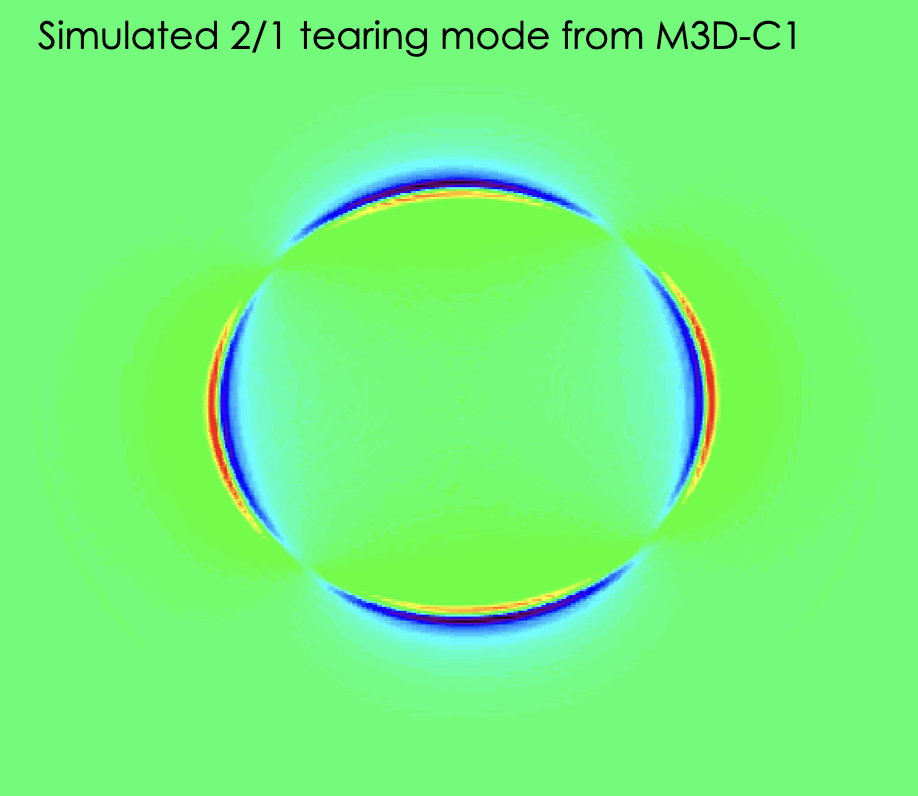
The culmination of this project will be the launch and analysis of the tearing mode data-base (TMDB) – a publicly available, DoE-sponsored database to study ML-accelerated tearing stability with a focus on tearing onset – in connection with the project on “Open and FAIR Fusion for Machine Learning Applications”. Community involvement will be sought in the application of exotic stability terms. The database will start with DIII-D data, and expand to C-Mod and beyond.
The tearing-free scenario team is led by graduate student Stuart Benjamin (MIT Physics). Collaborators include Ryan Sweeney (CFS), Francesca Turco (Columbia U.), Laszlo Bardoczi (UC Irvine), Jim Callen (UW Madison), and Arunav Kumar and Gregorio Luigi Trevisan (MIT). Stuart’s supervisors are Dr. Cristina Rea, Dr. Cesar Clauser, and Dr. Earl Marmar.